August 10, 2022
Amazon and University of Washington announce inaugural Science Hub faculty research awards
Six UW professors will advance artificial intelligence and robotics research with new grants.
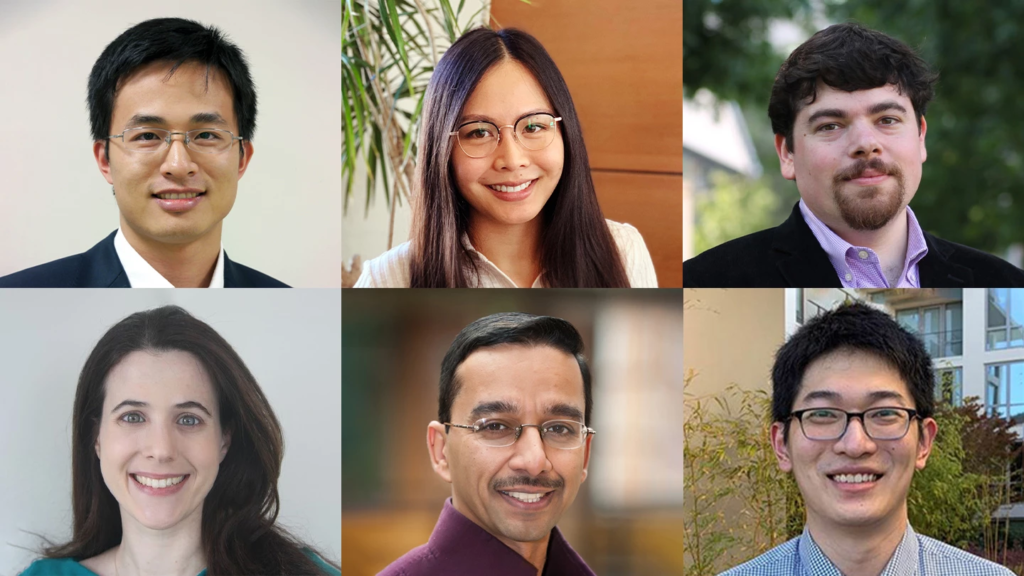
The UW + Amazon Science Hub, founded in February 2022 and housed in the University of Washington College of Engineering, has announced the recipients of its inaugural set of faculty research awards to advance artificial intelligence (AI) and robotics.
The projects were selected through a joint review process between the UW Advisory Group and Amazon. Each recipient will each receive up to $100,000 in research funding from Amazon, and each year-long project will address a real-world, cutting-edge challenge in AI or robotics.
Learn more about this year’s recipients below.
Adaptive Grasping and Object Manipulation using Visual and Tactile Feedback
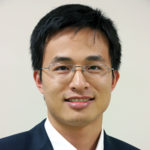
Xu Chen, McMinn Endowed Research Professor of Mechanical Engineering
The project aims to enable industrial collaborative robots with the manipulation intelligence that humans employ to grasp and manipulate objects with heterogeneous feedback. Humans use a combination of visual and tactile sensing to grasp and manipulate objects. Previously, numerous studies have proposed purely visual or purely tactile feedback algorithms to grasp objects. With recent advances of perception, computation, and sensor fusion, this project will integrate visual and tactile feedback to grasp and manipulate objects. The geometry, material and loading of the objects will not be known a priori. The study will use a UR5e robot fitted with a 2D stereo camera and a parallel gripper with pressure sensors for experimental validation of grasping and manipulation algorithms.
Shifting From Reactive to Proactive Safety: Legible Contingency Planning for Prosocial Interactions
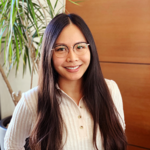
Karen Leung, assistant professor, aeronautics and astronautics
The goal of this project is to innovate towards a proactive safety framework for robot planning and control in multi-agent interactive warehouse navigation settings, a stark departure from typical reactive safety paradigms. The key insight is to develop legible robot motion to induce prosocial human-robot behaviors (i.e., taking actions to benefit the group), resulting in (i) safe and seamless human-robot interactions, and (ii) a reduction in the frequent use of reactive safety controllers which degrade performance and may damage the robot or cargo.
Dynamic Stiffness for Rapid Gripping Using Metamaterials
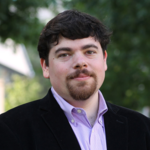
Jeffrey Lipton, assistant professor of mechanical engineering
Vacuum adhesion using suction cups is a vitally important method for grasping objects in an Amazon warehouse. These systems use extending rods to move the cups away from an industrial robotic arm’s wrist down to the target object. This causes two problems: firstly, the rigid connection between the object and arm makes the suction cups susceptible to pealing and secondly it requires the large arms to gimbal to pick up items. This gimbaling motion takes up space and slows down the picking and stowing process. We will solve these problems by generating a new type of end effector based on mechanical metamaterials known as handed shearing auxetics (H.S.A.). H.S.As are patterns on hollow tubes that can convert rotation directly into extending or bending movements and can dynamically change stiffness. We will develop a rapidly articulable wrist and validate it on grasping tasks to compare the pick time and space used with those of a traditional robot arm. Next, we will learn to use the dynamic stiffness of the H.S.A to perform picking operations with higher reliability. Finally, we will develop an extendable flex shaft for driving multistage H.S.A. systems. Longer term this will lay the foundation for low-cost and safe arms made entirely from metamaterials for picking and stowing tasks.
Design-Aware 3D Scene Interpretation
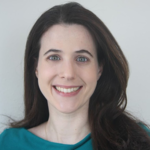
Adriana Schulz, assistant professor of computer science and engineering
Though image-based recognition and reconstruction is a well-studied problem, recovering an accurate 3D model from images remains challenging, particularly for scenarios with clutter and occlusions. Typical methods rely on retrieval from a database and are intractable when 3D models of the items are not available. In this proposal, we uniquely observe that manufacturability defines and constrains the design space for man-made objects and can be leveraged to develop novel reconstruction methods. Using design for manufacturing as an underlying representation, we can reduce the search space to models that can be represented in Computer-Aided-Design (CAD) systems, making the inverse reconstruction problem easier to manage. We demonstrate how this approach can improve local precision in 3D reconstruction to enable robust robotic manipulation.
Self-Supervised Learning of Part-Whole Hierarchies for Semantic Scene Understanding, with Applications to Representing Densely Packed Bins and Mobile Robotics
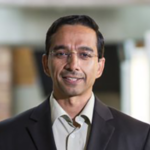
Rajesh P. N. Rao, CJ and Elizabeth Hwang Professor of Computer Science
A key problem in automating the semantic understanding of objects, scenes and environments is learning compositional, part-whole representations from images and videos. We propose a new deep learning framework called Active Predictive Coding Networks (APCNs) for solving this important problem. Inspired by emerging ideas in neuroscience and cognitive science, APCNs utilize hierarchical reference frames and action-conditional predictions to learn versatile spatiotemporal representations of a scene that are compositional, generative, and probabilistic. By combining reinforcement learning and active inference for inferring actions with self-supervised learning of interpretable world models, APCNs lend themselves naturally to applications such as representing and manipulating densely packed bins and modeling the dynamically changing environments of mobile robots.
Fleet Planning of Autonomous Cart Systems in Modern Fulfillment Centers
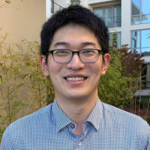
Chiwei Yan, assistant professor, industrial and systems engineering
Modern fulfillment centers are replacing traditional conveyor belt systems, forklifts or automated guided vehicles with multi-purpose autonomous carts. These autonomous carts are designed to work alongside human and automatically transport stock keeping units (SKUs) from an origin location to a destination location, without following a fixed path, which allows them to be flexibly deployed in existing facilities without significant infrastructure changes. This proposal concerns the fleet planning problem of deploying such autonomous cart systems in practice — how many carts are needed to achieve certain throughput rate given the layout and service capacity of the facility, and what are the resulting key performance measures such as cart utilization rate and waiting times. This decision is complex because of a multitude of conflicting factors such as the dichotomy of cart density on service availability and congestion, which requires dedicated analytics capabilities. We propose an array of novel, simple and practical models that can guide practitioners to a reliable initial estimate of fleet sizing, before running expensive field experiments or building customized simulation software.
The UW + Amazon Science Hub also supports doctoral fellowships and sponsors longer-term research and development aligned with Amazon interests. For more information on events and activities, visit the official site.