January 7, 2025
UW + Amazon Science Hub announces 2024-25 Amazon Fellows and Faculty Research Awards
The UW + Amazon Science Hub, established in 2022 to advance fundamental research and applications of robotics and artificial intelligence, has announced its 2024-25 Amazon Fellowships and Faculty Research Awards.
Amazon Fellows
2024-25 Amazon Fellowships were awarded to three UW Engineering doctoral students. Amazon Fellows receive three quarters of funding to pursue independent research projects, as well as opportunities for paid summer internships at Amazon, where they can work directly with Amazon researchers to gain valuable industry insight and experience.
Ainaz Eftekhar
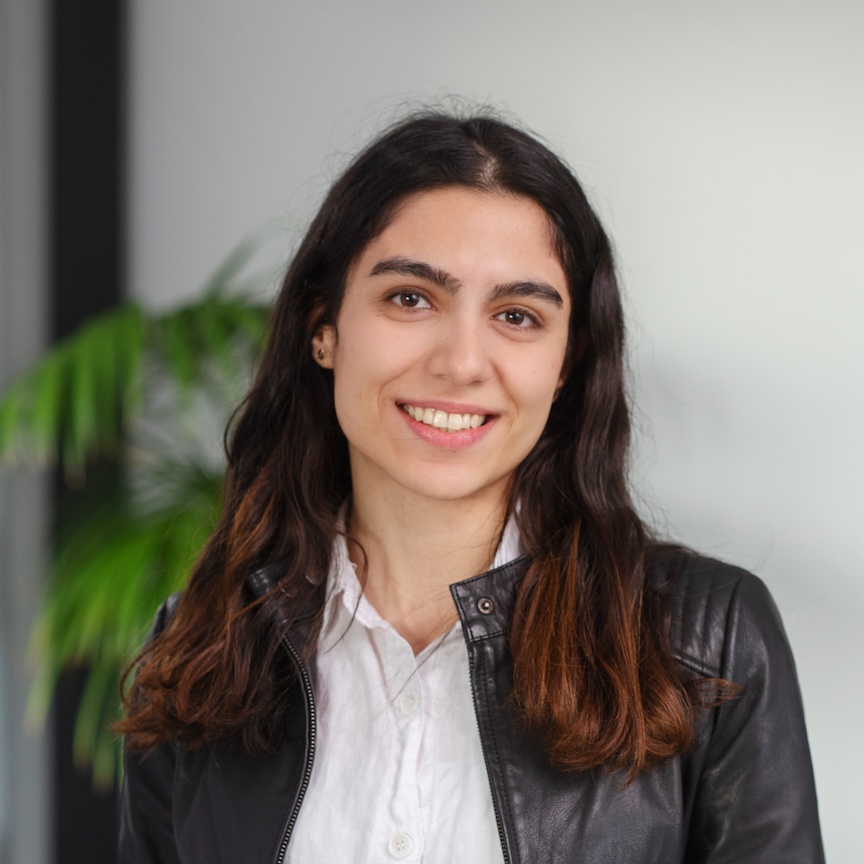
Ainaz Eftekhar is a Ph.D. student in computer science and engineering (CSE) and a member of the RAIVN lab, advised by Ali Farhadi and Ranjay Krishna. Her research focuses on the intersection of computer vision, machine learning, and Embodied AI, where she develops algorithms that enable robotic systems to perceive and intelligently interact with their environments.
A Generative Approach to Co-optimization of Morphology and Control
A robot’s functionality is highly influenced by its design. In industrial applications, task-specific designs are essential for ensuring that the system is robust — that it can handle a range of variables affecting that task. However, manual design is costly, time-consuming, and heavily reliant on an engineer’s expertise.
This project proposes a generative approach to robot design: automating the process of searching the design space to discover the optimal structure and control system for a robot to best perform a specific task.
Jake Gonzales
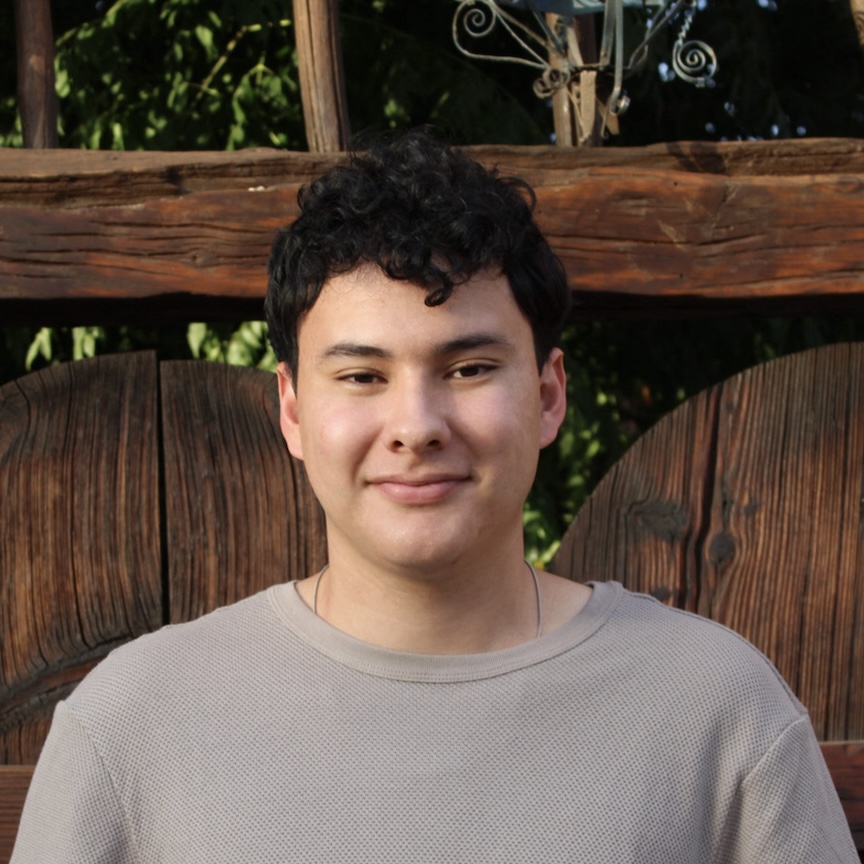
Jake Gonzales is a Ph.D. student in the department of electrical and computer engineering and a member of the Autonomous Controls Lab, co-advised by Behçet Açıkmeşe and Lillian Ratliff. His research lies at the broad intersection of control theory, machine learning and AI, optimization, and game theory. His current work focuses on developing methodologies and decision-making algorithms that enable safe and scalable learning-enabled autonomous systems to operate reliably in uncertain real-world environments.
Foundation Models for Scalable Decision-Making in Multi-Agent Autonomous Mobility
This research addresses the challenges of large-scale multi-agent autonomous mobility in congested, shared environments. Current methods, such as search-based algorithms, often struggle to scale efficiently and handle non-stationarity arising from agent interactions in dynamic, uncertain settings. While RL has shown promise in robotic tasks, it traditionally requires extensive training data for each specific task, limiting its effectiveness in data-scarce environments. We propose leveraging foundation models (FMs), pretrained on vast multimodal datasets, to improve decision-making in autonomous mobility. We will integrate FMs into multi-agent RL algorithms, creating a general algorithmic framework that can be fine-tuned across diverse downstream tasks. This integration aims to improve data efficiency in learning effective predictors for controllers and robot path planning policies. Next, we will refine FMs using principled game-theoretic abstractions, such as Stackelberg games, by casting them as strategic decision-making agents. This approach will enable task-relevant knowledge extraction through repeated interactions, optimizing performance for specific tasks with the aim of creating efficient, domain-specific models suitable for real-time deployment in robot planning. Finally, focusing on the specific application of path planning and task assignment of thousands of autonomous robots in warehouses, we will utilize the multimodal representation capabilities of FMs to enhance learning of complex congestion patterns, facilitating more effective state space exploration and adaptation to dynamic environmental conditions. The expected outcomes are principled algorithms with theoretical guarantees, validated through large-scale simulations. We plan to augment existing public datasets with synthetically generated data and deploy the developed methods on real-world ground robots to demonstrate practical applicability.
Kazuki Mizuta
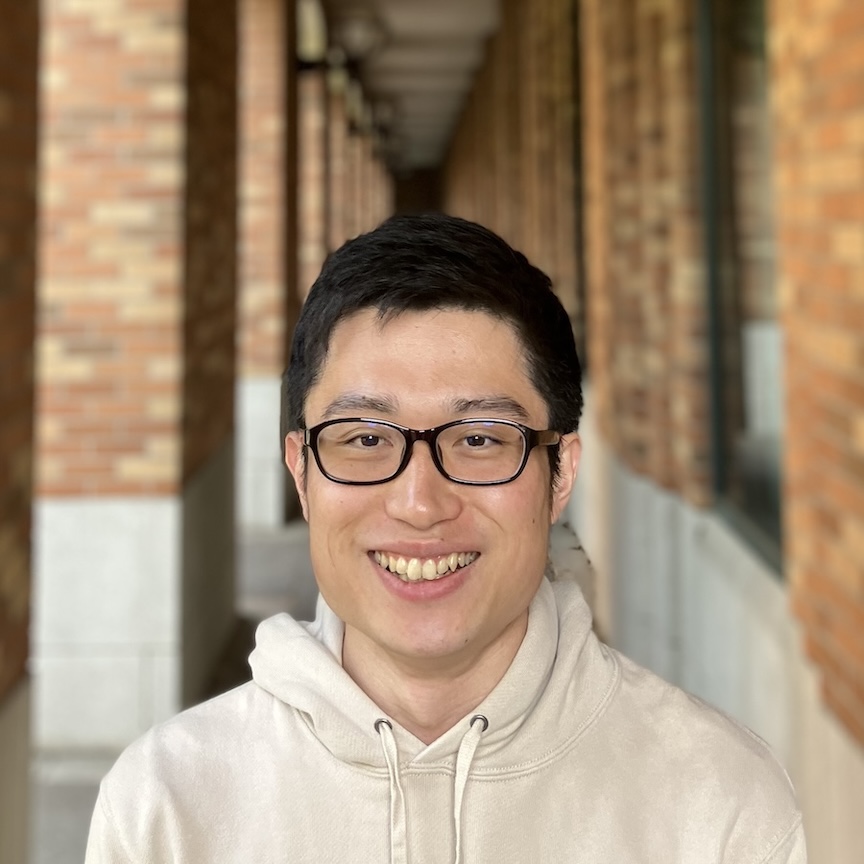
Kazuki Mizuta is a Ph.D. student in Aeronautics and Astronautics (A&A) and a member of the Control and Trustworthy Robotics Lab, advised by Karen Leung. His research revolves around the intersection of machine learning and control theory, enabling autonomous mobile robots to achieve safe and interpretable behavior in uncertain and dynamic safety-critical environments.
Towards Safe and Predictable Social Navigation for Autonomous Ground Vehicles
Autonomous ground vehicles (AGVs) have the potential to improve productivity, accessibility, and human safety in various applications, including heavy load transport, package delivery, robot taxi, and environmental monitoring in both indoor and outdoor settings. To achieve human trust and widespread adoption, not only must AGVs demonstrate an ability to navigate safely in shared human spaces, they must navigate predictably.
This project aims to develop a real-time robot planning algorithm that synthesizes safe and predictable movements in dynamic environments. The approach involves integrating the expressiveness and flexibility of generative models with systems that provide interpretable safety controls.
Faculty Research
2024-25 Faculty Research Awards support five new research projects that include seven UW faculty. Each project receives up to $100,000 in support from Amazon.
Adversarial Object Generation for Robust Manipulation
Despite the recent surge of progress in generative AI, practical applications such as robotic manipulation of objects require large-scale training data and systems that effectively adapt to new tasks. This project aims to address both issues by training a generative model to simulate additional 3D training data, and by creating a game between that model, simulating increasingly challenging new objects, and the robot manipulation system, learning to handle them. This “adversarial co-training” approach aims to advance a robot manipulation system that can handle the massive, diverse, and constantly changing distribution of products in Amazon warehouses.
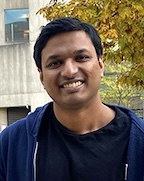
Assistant Professor of Computer Science & Engineering
Combining Physics-Based and Learned Models in Hybrid Simulators for Robotic Manipulation
The goal of this project is to advance robotic manipulation systems by researching fundamental techniques in combining machine learning algorithms with physics-based simulations of objects. Hybrid models will enable robots to more accurately and efficiently render a much larger class of objects in warehouse environments for tasks like stowing and unstowing objects.
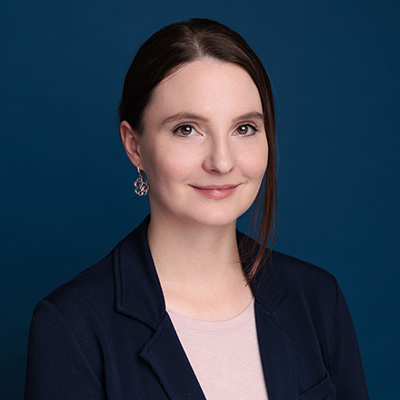
Assistant Professor of Computer Science & Engineering
Efficient Manipulation in Presence of Dynamic Uncertainties Via Output-Sampled Model-Based Learning Control
If human workers and robots interact in a shared workspace, real-time perception and motion planning systems are crucial for robots to cope with dynamic situations.
Despite numerous advances in motion planning, machine learning, and sampling-based algorithms, existing robotic manipulation systems struggle to adapt to new objects and changing environments, particularly as robots gain complexity and range of motion.
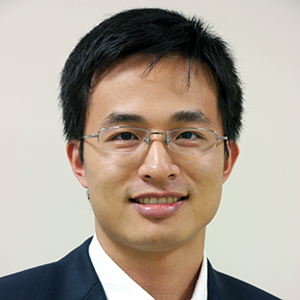
Associate Professor of Mechanical Engineering
Bryan T. McMinn Endowed Research Professorship
Director of the Boeing Advanced Research Collaboration (BARC)
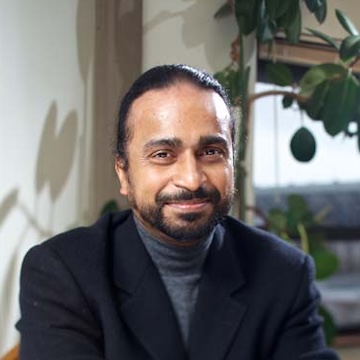
Minoru Taya Endowed Chair, Mechanical Engineering
This project aims to develop more efficient robotic manipulation systems that can handle more complex tasks. The researchers aim to foster a step change in sampling-based algorithms by using a technique known as “model inversion” along with perceptual data from multiple sensors.
Leveraging the Common Sense of Large Language Models for Robotic Manipulation
This project aims to leverage the ability of large language models (LLMs) to capture and distill “human common sense” to help explain, correct, and improve physical manipulation tasks. The ultimate goal of this research is to be able to point a camera at an operation in a warehouse and fine-tune an LLM that is capable of performing the same kind of common-sense inference as a person watching that operation.
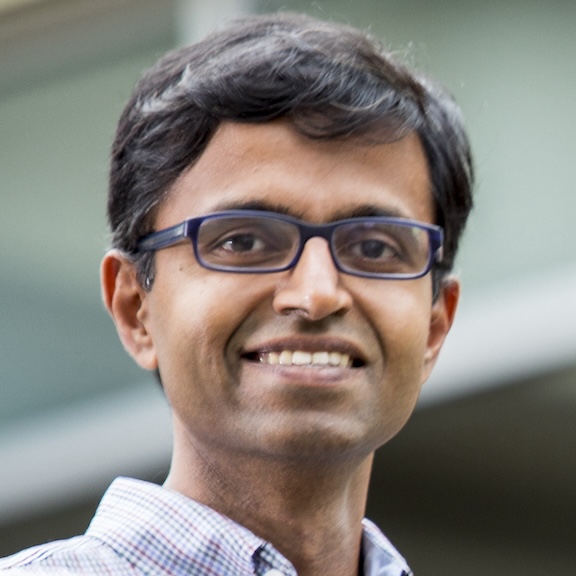
Professor of Computer Science & Engineering
Smart Suction Cups Enabled by Electrostatic Sensing and Actuation
This project aims to advance robotic manipulation by developing biologically inspired “smart” suction cups that include sensors and actuators. Recent advances in 3-D printing enable the production of industry-compliant soft suction cups that incorporate electromechanical robot technologies. If successful, this research will improve the grasp success rate and picking velocity (units per hour) of robots.
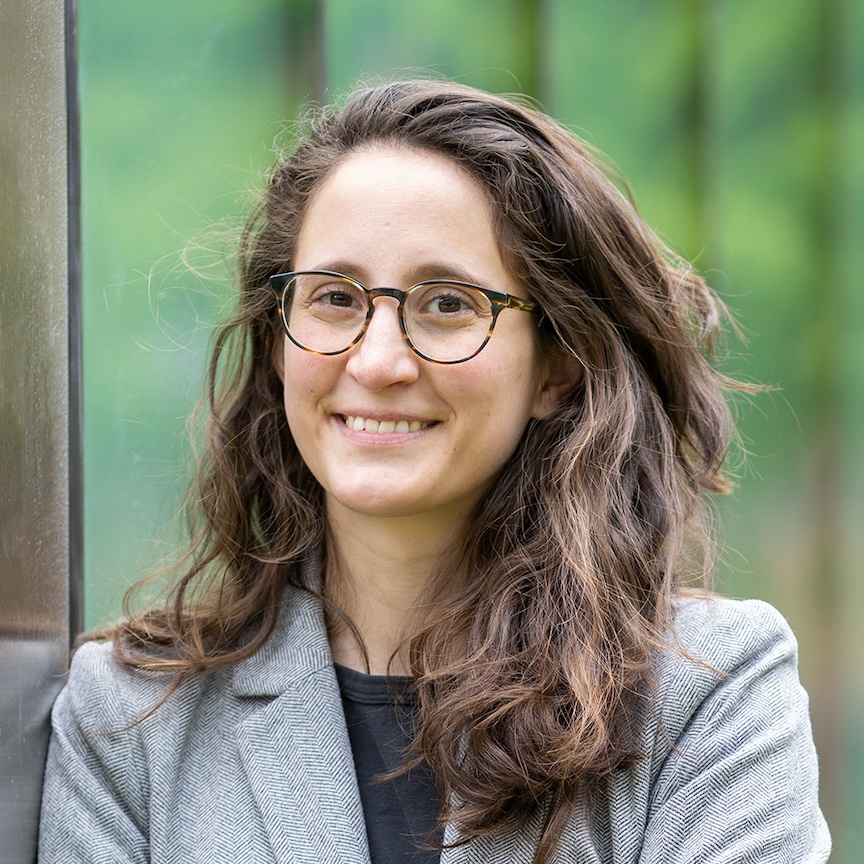
Assistant Professor of
Electrical & Computer Engineering
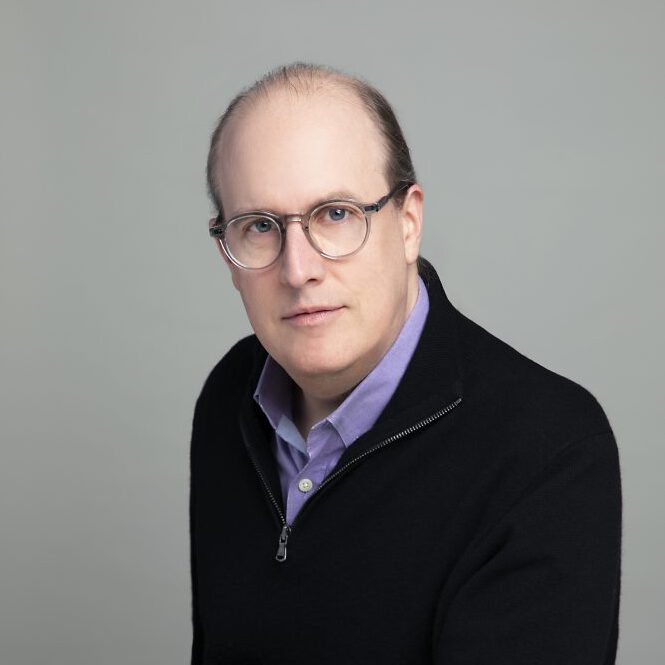
Milton and Delia Zeutschel Professor in Entrepreneurial Excellence, Computer Science & Engineering and Electrical & Computer Engineering
Director of the UW + Amazon Science Hub